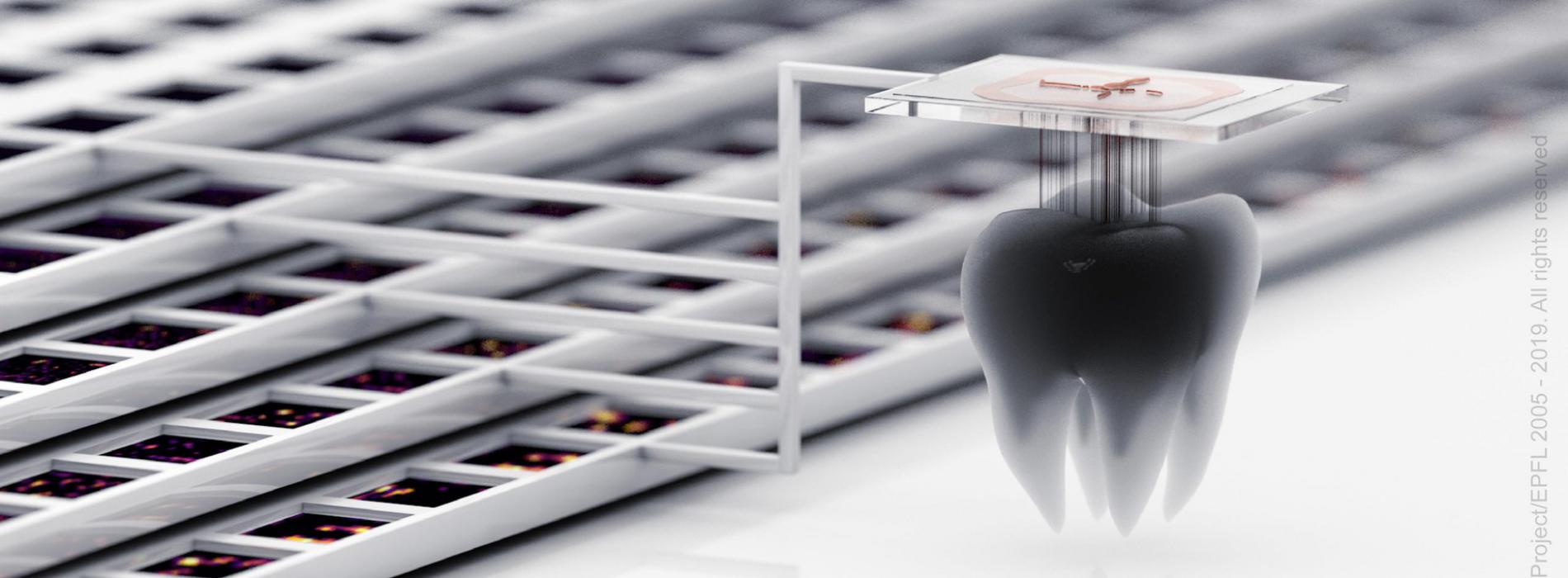

A deep learning model for early tooth decay detection
Tooth decay (dental caries) is a major global public health problem and according to the WHO is the most widespread non-transmissible disease in the world. Transillumination with near-infrared light imaging (TI) has been shown to be effective in the vital early detection of tooth decay. Applying translational research and technology, scientists from the EPFL Blue Brain Project and the University of Geneva have collaborated to develop a new deep learning model for the automated detection and localization of dental lesions in TI images.
The WHO also notes that tooth decay was the most prevalent condition included in the 2015 Global Burden of Disease Study, ranking first for adult teeth decay (2.3 billion people) and 12th for milk teeth (560 million children). Severe dental caries can impair a person’s quality of life, including difficulties in eating and sleeping, and in its advanced stages (abscesses), can result in pain and chronic systemic infection.
Early indications of the decay process are small patches of demineralized (softened) enamel at the tooth surface, often hidden from sight in the fissures (grooves) of teeth or in between the teeth. The destruction spreads into the softer, sensitive part of the tooth beneath the enamel. The weakened enamel then collapses to form a cavity and the tooth is progressively destroyed. Caries can also attack the roots of teeth if they become exposed by gum recession.
The main treatment option for a tooth cavity is to drill out the decay and put in a filling. Extensive tooth decay can involve a variety of different treatments or even removal of the tooth. Therefore, early detection is vital as it can significantly improve treatment outcomes and reduce the need for invasive procedures.
Deep learning model for the automated detection and localization of dental lesions
As a result of its commitment to the pursuit of novel and innovative strategies for multi-scale, data-driven brain simulations and utilizing biologically detailed digital reconstructions (computer models) to explore function and dysfunction in brain tissue, the Blue Brain has gained new and important insights into the functional and structural connectivity of neural circuits. These insights can be leveraged in the development of machine learning software to process and/or classify images.
During their research into prevention and treatment of dental injuries caused by dental decay, the University of Geneva has collected a dataset of medical images comprising dental scans of human teeth, including teeth exhibiting carious lesions. These datasets are suitable for training and validating machine learning for the detection of dental disease and with transillumination with near-infrared light (TI) imaging being shown to be effective for the detection of early stage lesions, the potential to collaborate by bringing together both parties resources and expertise was identified.
In a paper published in the Journal of Dental Research, scientists at the Blue Brain Project and the University of Geneva have developed a deep learning model for the automated detection and localization of dental lesions in TI images. Based on a convolutional neural network trained on a semantic segmentation task, the method uses various strategies to mitigate issues related to training data scarcity, class imbalance, and overfitting. To complement this, each pixel is predicted as being background, dentin, enamel, occlusal caries, or proximal caries.
To date, previous efforts documented in the literature suffered from low accuracy, required hundreds to thousands of samples for training, or solved a simplified problem.
“With only 185 training samples, our model achieved an overall mean intersection-over-union (IOU) score of 72.7% on a 5-class segmentation task, and specifically an IOU score of 49.5% and 49.0% for proximal and occlusal carious lesions, respectively,” explains Francesco Casalegno, Blue Brain’s Machine Learning Section Manager.
“This represents a dramatic improvement on previous efforts that obtained an IOU of 7.5% for carious lesions using X-ray images. In addition, we constructed a simplified task, in which regions of interest were evaluated for the binary presence or absence of carious lesions (area of decay on the tooth crown or root). For this task our model achieved high scores for the area under the receiver operating characteristic curve of 83.6% and 85.6% for occlusal and proximal lesions, respectively.”
Results hold promise for increasing the speed and accuracy of tooth decay detection
These results demonstrate that a deep learning approach for the analysis of dental images holds much promise for increasing the speed and accuracy of caries detection, supporting the diagnoses of dental practitioners, and improving patient outcomes. They also suggest that a similar approach could be used for the automated interpretation of DIAGNOcam® (infrared radiation for dental transillumination) scans to facilitate dental diagnoses.
Click here to read the paper - https://doi.org/10.1177/0022034519871884
Casalegno, F., Newton, T., Daher, R., Abdelaziz, M., Lodi-Rizzini, A., Schürmann, F., Krejci, I., and Markram, H. (2019). Caries Detection with Near-Infrared Transillumination Using Deep Learning. Journal of Dental Research. Online 26 August 2019.
For more information, please contact Blue Brain Communications Manager – kate.mullins@epfl.ch
https://actu.epfl.ch/news/a-deep-learning-model-for-early-tooth-decay-dete-2/